Okay, here’s an Overview section designed to meet those specifications:
Overview: Data Mining’s Dark Side: How It’s Reshaping (and Exploiting) Big Data
The Ubiquitous Landscape of Data Mining
The modern business landscape is inextricably linked to data. Massive datasets, often referred to as ‘Big Data,’ are now the lifeblood of organizational decision-making, powering everything from targeted marketing campaigns to complex risk assessments. At the heart of this data-driven revolution lies data mining, the sophisticated process of uncovering patterns, trends, and insights from these vast repositories of information. This powerful analytical approach enables organizations to gain a competitive edge, optimize operations, and personalize customer experiences. However, the immense capabilities of data mining also harbor a less explored, and potentially problematic, side.
The Double-Edged Sword of Data Insights
While data mining offers unparalleled opportunities for progress and innovation, its power can also be misused. The very mechanisms that enable positive transformations – complex algorithms, automated pattern recognition, and predictive analytics – can also be leveraged for manipulative, discriminatory, or unethical purposes. This blog post delves into these less-discussed aspects, examining how data mining’s inherent biases, potential for privacy violations, and susceptibility to misuse are reshaping the Big Data landscape. The goal is to provide a critical analysis for data mining professionals and business leaders, empowering them to navigate the ethical complexities inherent in this powerful technology.
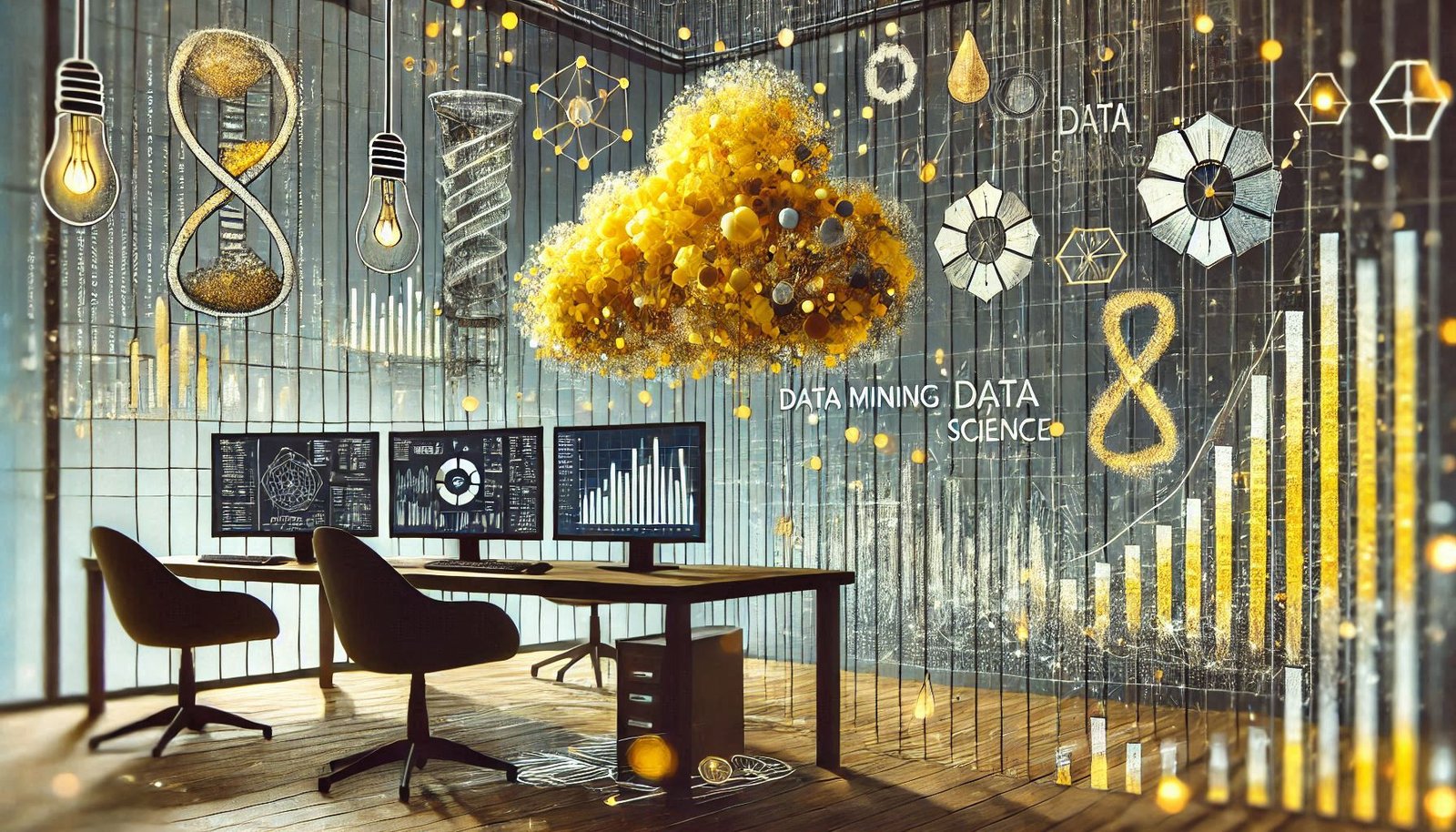
Key Areas of Exploration
This discussion will analyze:
- Algorithmic Bias: How unintended prejudices can be embedded within data and amplified through data mining processes, leading to unfair or discriminatory outcomes (O’Neil, 2016).
- Privacy Concerns: The erosion of individual privacy stemming from the widespread collection and analysis of personal data, including the challenges associated with anonymization and data breaches.
- Exploitation of Behavioral Patterns: The potential for data mining to be used to subtly manipulate consumer behavior and exploit vulnerabilities.
- Ethical Considerations and Regulatory Frameworks: Examining the need for proactive ethical guidelines and robust regulatory frameworks to ensure responsible data mining practices.
By exploring these critical issues, this blog aims to foster a deeper understanding of both the benefits and the potential pitfalls of data mining, encouraging more responsible and transparent use of these transformative technologies. (Zuboff, 2019).
References:
- O’Neil, C. (2016). Weapons of math destruction: How big data increases inequality and threatens democracy. Crown.
- Zuboff, S. (2019). The age of surveillance capitalism: The fight for a human future at the new frontier of power. PublicAffairs.
Okay, here’s an analysis of the data mining market, focusing on key trends, their impact, and actionable insights:
Analysis of the Data Mining Market: Trends, Impact, and Actionable Insights
The data mining market, encompassing tools and techniques for extracting valuable insights from large datasets, is experiencing dynamic shifts. This analysis will categorize these trends, assess their impact, and provide actionable insights for strategists in the field.
Positive Trends
- Democratization of Data Mining Tools: There’s a significant movement towards user-friendly, low-code/no-code platforms. These tools are simplifying complex algorithms, making data mining accessible to a wider range of professionals beyond specialized data scientists.
- Underlying Factor: Increased demand for data-driven decision-making across various departments and organizations coupled with a shortage of skilled data scientists.
- Impact: Reduced dependency on specialized personnel, accelerated insight generation, and increased adoption by SMEs. Example: Tableau and Alteryx are popular tools enabling business users to perform data analysis.
- Actionable Insight: Invest in user training programs and low-code platforms to empower employees across various departments to extract insights and foster a data-driven culture.
- Rise of Cloud-Based Data Mining: The adoption of cloud-based platforms for data mining is increasing rapidly. Cloud environments provide scalable resources, reducing the need for costly on-premise infrastructure.
- Underlying Factor: Cloud computing offers cost-effectiveness, agility, and the ability to handle massive datasets. It also allows for easier collaboration and data sharing.
- Impact: Reduced infrastructure costs, increased accessibility to advanced algorithms, and faster deployment of data mining models. Example: Google Cloud AI Platform and Amazon SageMaker offer robust cloud-based data mining solutions.
- Actionable Insight: Strategically migrate data and data mining operations to the cloud, optimizing for cost-effectiveness and scalability.
- Focus on Explainable AI (XAI): There’s a growing need for transparency and interpretability of AI and data mining models. XAI addresses the “black box” problem by making model decision-making processes understandable.
- Underlying Factor: Increased regulation, ethical considerations, and the need to build user trust in AI-driven outcomes.
- Impact: Increased user confidence, better model validation, easier detection of bias, and enhanced compliance. Example: LIME and SHAP are XAI methods used to explain complex models.
- Actionable Insight: Prioritize XAI tools and techniques when implementing data mining solutions, especially in sensitive domains like finance and healthcare.
Adverse Trends
- Data Privacy and Security Concerns: Growing concerns about data privacy and stringent regulations like GDPR and CCPA pose significant challenges. Companies must comply with these regulations while continuing to leverage data for insights.
- Underlying Factor: Increased awareness of data breaches, misuse of personal information, and growing consumer demand for data privacy.
- Impact: Increased costs associated with compliance, reputational risks associated with data breaches, and potential limitations on the use of certain data. Example: Companies facing fines and public backlash for violating GDPR.
- Actionable Insight: Invest in robust data security infrastructure and implement privacy-enhancing technologies (e.g., anonymization, differential privacy). Develop a comprehensive data governance framework that aligns with regulations.
- Data Quality Issues: The proliferation of data from various sources often leads to inconsistencies, incompleteness, and inaccuracies. Poor data quality can significantly impact the accuracy of data mining models.
- Underlying Factor: Rapid data growth, disparate data sources, and lack of standardized data collection processes.
- Impact: Misleading insights, biased predictions, and potential damage to decision-making. Example: A machine learning model trained on biased data may perpetuate inequalities.
- Actionable Insight: Invest in data quality management tools and processes, including data cleansing, validation, and monitoring. Adopt data governance policies to ensure data accuracy and consistency.
- Talent Gap in Advanced Analytics: While low-code platforms simplify data mining, there’s still a shortage of professionals skilled in advanced analytics, especially in areas like machine learning and deep learning.
- Underlying Factor: The rapid growth of the data mining field and the evolving complexity of algorithms are outpacing the development of specialized talent.
- Impact: Limited ability to leverage advanced analytics for competitive advantage, and potential delays in project implementation.
- Actionable Insight: Partner with academic institutions to foster talent development, offer competitive compensation packages, and invest in upskilling existing employees.
Conclusion
The data mining market presents both opportunities and challenges. By understanding these key trends, businesses can strategically invest in areas like user-friendly platforms, cloud infrastructure, and explainable AI, while simultaneously mitigating the risks posed by data privacy concerns, data quality issues, and talent gaps. The key to success lies in proactively adapting to the changing landscape and making data-driven strategies a core component of business operations.
### Real-Life Applications of Data Mining in Business
Healthcare: Data mining is crucial for improving patient care and optimizing operations. Hospitals use it to predict patient readmission rates by analyzing historical data like medical history, demographics, and treatment details. Identifying patients at high risk allows for proactive interventions, such as enhanced discharge planning or follow-up care, thereby reducing costs and improving outcomes. Pharmaceutical companies also employ data mining to accelerate drug discovery by analyzing genetic data to identify potential drug targets and predict drug efficacy using clinical trial data. This speeds up the drug development process and reduces the likelihood of failed clinical trials.
Technology: Tech companies heavily rely on data mining for personalization and product development. E-commerce platforms use association rule mining to identify items frequently purchased together, enabling them to offer tailored recommendations to customers. This improves customer experience and drives sales. Furthermore, social media companies leverage sentiment analysis—a data mining technique—to understand user opinions about products, brands, or news. This provides valuable insights for targeted advertising campaigns and product enhancements. Companies also use pattern analysis to find anomalies in network traffic, helping them to quickly detect and respond to cybersecurity threats, like malware attacks, and protect sensitive user data.
Automotive: The automotive industry employs data mining for vehicle maintenance and product improvement. Manufacturers analyze sensor data from cars to predict when parts might fail, allowing them to proactively schedule maintenance for optimal vehicle performance and to minimize breakdowns. This predictive maintenance also saves resources, as technicians will focus on specific maintenance, and improve car owners’ experience by reducing inconvenient repairs. Additionally, data analysis on driving behavior helps companies improve vehicle design based on real-world usage patterns. For instance, engineers can identify areas where cars tend to break down, which can inform materials and design decisions of future models.
Manufacturing: Manufacturers use data mining to optimize production processes and enhance quality control. By analyzing sensor data from machines, companies can detect potential equipment failures before they occur, reducing downtime and maintenance costs. This predictive maintenance approach helps maintain an efficient production line and prevent costly repairs. Moreover, by looking at the root cause of defects in production lines, they can optimize each step of the process, reduce the number of defective products, and improve the overall quality of the goods.
### Key Strategies in the Data Mining Space (2023 Onwards)
Organic Strategies:
- Focus on Explainable AI (XAI): Companies are increasingly investing in developing data mining tools that offer more transparent and interpretable results. Instead of just providing predictions, they aim to explain why those predictions were made. This builds trust with users and ensures better compliance with regulatory requirements. For example, a fraud detection company might now offer visualizations showing exactly which factors triggered an alert, rather than just a generic “suspicious” flag.
- Enhanced Automation and MLOps: There is a strong push to automate various stages of the data mining pipeline, from data preparation to model deployment. This involves investing in Machine Learning Operations (MLOps) platforms that streamline the development, testing, and monitoring of data mining models. A company selling marketing analytics solutions, for example, is now offering self-service tools for building customer segmentation models, which requires minimal coding knowledge from the end user.
- Real-time Data Processing & Analytics: More companies are focusing on providing data mining solutions that can process and analyze data in real-time or near real-time. This is driven by the increasing demand for immediate insights from streaming data sources like IoT devices or social media feeds. For instance, a supply chain management company now has capabilities to monitor real time sensor readings and immediately take actions if an anomaly is detected in warehouse temperatures.
Inorganic Strategies:
- Strategic Acquisitions of Niche Technology Providers: Companies are acquiring smaller businesses with specialized data mining expertise to quickly expand their product portfolios or enter new markets. For instance, a large cloud provider could acquire a company specializing in graph database technology to enhance its data mining capabilities for connected data.
- Partnerships and Alliances: Collaboration with other tech companies, research institutions, or industry-specific data providers is a growing trend. These partnerships can allow access to unique data sources, or provide technology know-how to enhance existing platforms. An example would be a healthcare data mining company forming an alliance with a genetics research center to improve its disease prediction models.
- Investment in Open Source Initiatives: Many organizations are contributing to and building on open-source data mining projects. This fosters innovation and attracts talent while also reducing development costs. A financial services firm could actively contribute to a popular data visualization framework to gain a competitive edge by creating unique charts that are available to everyone.
Okay, here’s an Outlook & Summary section designed to meet those requirements:
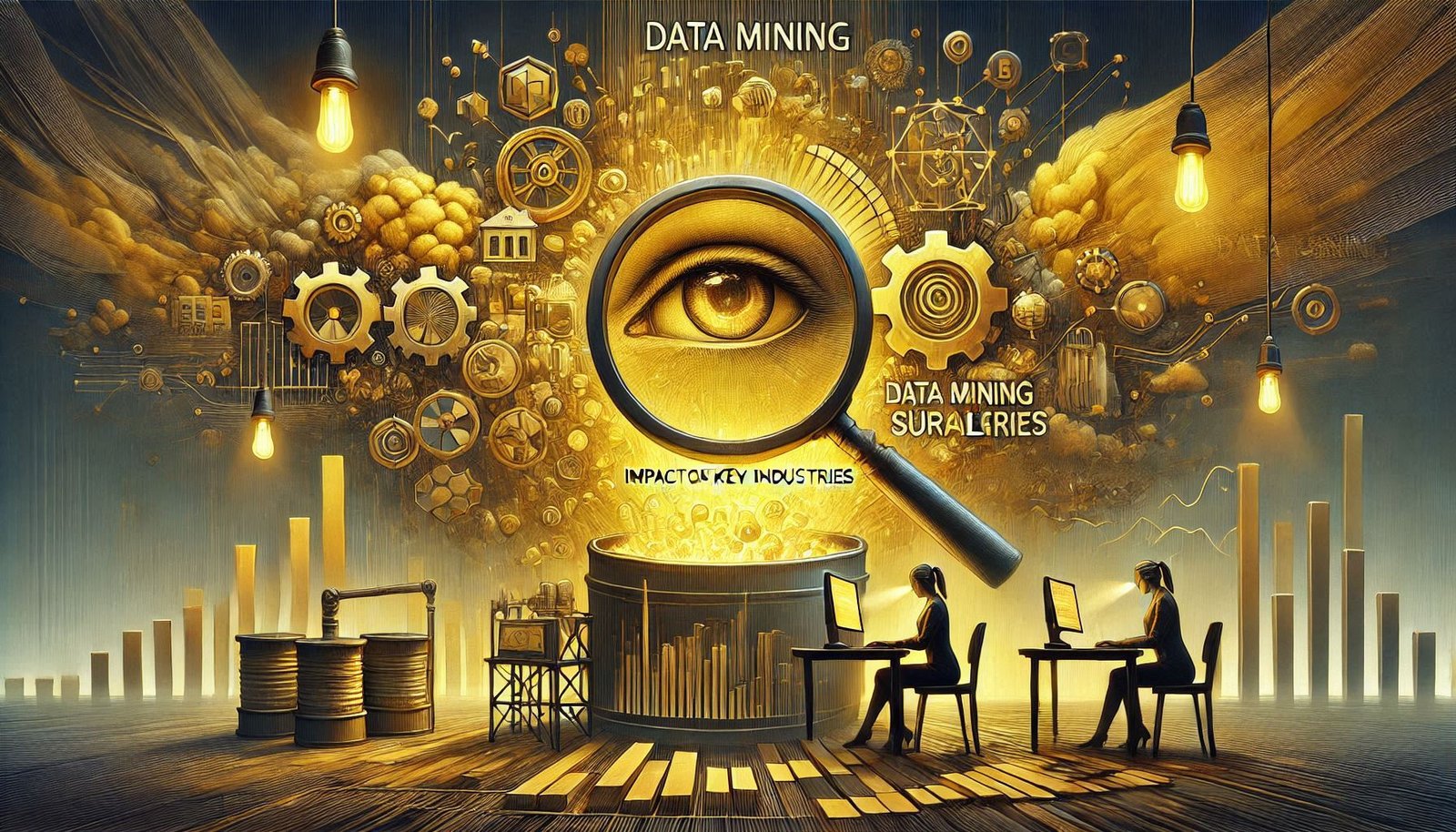
Outlook & Summary: Navigating the Evolving Landscape of Data Mining
Future Trajectories: 5-10 Years Out
The next decade will likely see data mining become increasingly sophisticated, driven by advancements in artificial intelligence and machine learning. We can anticipate:
- Enhanced Automation: A greater reliance on automated data mining processes, reducing the need for manual intervention. This includes improved feature selection and model development techniques.
- Ethical & Regulatory Scrutiny: Increased focus on the ethical implications of data mining, including bias detection and mitigation. Expect stricter regulations governing data use and privacy, impacting how data is mined and applied.
- Real-Time Insights: A shift towards real-time data analysis and mining, enabling businesses to make faster, more adaptive decisions. This will demand robust and scalable data infrastructure.
- Democratization of Tools: The evolution of user-friendly data mining platforms and tools, allowing more stakeholders within an organization to leverage its power, not just specialized analysts.
- Integration with Emerging Technologies: A closer integration of data mining with technologies like edge computing and the Internet of Things (IoT), opening new avenues for data collection and analysis.
Key Takeaways: The Double-Edged Sword
This article underscores a critical understanding: Data mining is not merely a technical process; it is a force that shapes the entire big data landscape. Its ability to extract valuable insights from massive datasets comes with the potential for misuse and exploitation. The core takeaway is that while data mining empowers businesses with unprecedented capabilities, it also brings serious ethical considerations and risks. Effective governance, ethical frameworks, and a clear understanding of these potential downsides are essential to ensure its responsible application. Data mining is an integral part of the big data ecosystem, and how we manage it, will greatly impact the efficacy and social acceptance of the entire sector.
Considering the ever-evolving nature of data mining and its far-reaching implications, how will your organization proactively address both the opportunities and challenges it presents to ensure ethical and effective use of big data?