Okay, here’s an attempt at that compelling overview section:
The air in the server room used to hum with a predictable, almost comforting monotony – the whirring of fans, the blink of status lights. It was a world of structured databases and rigid rules, a landscape I, like many of you, navigated for years. We built systems, maintained networks, and focused on keeping the digital lights on. But something shifted, a tectonic tremor rumbling beneath the surface. I remember the first time I saw a data visualization – a chaotic storm of points that coalesced into a clear, undeniable trend. It felt like peering through a fog, suddenly seeing the landscape laid bare. That was the whisper of the data science revolution, a quiet shift from reactive to predictive happening right under our noses.
Today, that hum has been replaced by a more complex symphony – the clatter of algorithms, the pulse of real-time analytics, the quiet intelligence extracted from oceans of data. The very foundation of IT is being redefined, not by more powerful hardware, but by the power of insight. We, the guardians of the technological realm, are now being asked to not just manage the flow of information, but to interpret its language. No longer is IT solely about what we do, but why and how it impacts the business in the long-run. We’re standing at the precipice of a new era. This silent revolution, powered by data, is not just changing our tools; it’s changing our roles. It’s reshaping the landscape of IT, and the opportunities – and challenges – are more profound than we might imagine. Are we ready to fully embrace it? Join me as we explore the transformative power of Data Science and navigate this new frontier.
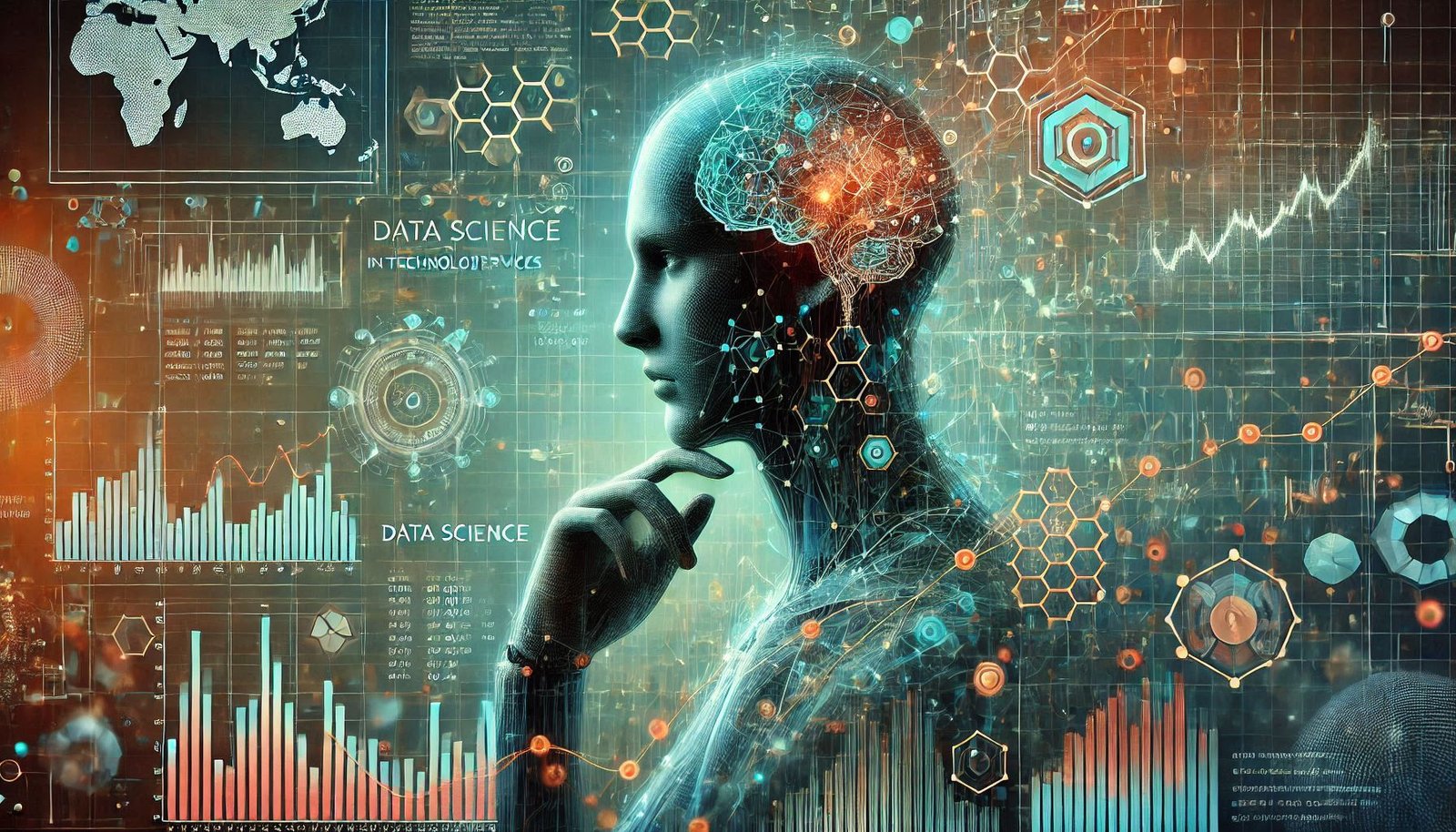
Okay, let’s dive into the fascinating, sometimes turbulent, world of Data Science, where numbers dance and algorithms whisper secrets. Imagine a bustling marketplace, overflowing with talent, tools, and possibilities – that’s the Data Science arena right now, and it’s changing faster than you can say “regression analysis.”
Positive Trends: The Rising Tide of Opportunity
First, let’s bask in the sunlight of Democratization of AI. Gone are the days when data magic was confined to wizard-like PhDs. Platforms like Google’s Vertex AI and Dataiku are putting powerful tools into the hands of citizen data scientists, empowering even small businesses to glean insights previously out of reach. Imagine a local bakery, once relying on gut instinct, now using predictive analytics to minimize waste and perfectly time their bread production. This isn’t just tech, it’s empowerment, painting a future where data-driven decisions become ubiquitous.
Then there’s the Explosion of Open-Source. Python, R, and a constellation of open-source libraries are now the lingua franca of data. The free flow of ideas and code is not just accelerating innovation; it’s creating a collaborative ecosystem where problems are solved collectively, like a group of artists contributing to a giant mural. Companies like Anaconda, thriving by curating these resources, demonstrate how collaboration fuels growth.
Adverse Trends: Navigating the Murky Waters
However, not all is rosy. The Talent Crunch remains a formidable obstacle. Finding experienced data scientists is akin to searching for a specific snowflake in a blizzard. This intense competition drives up salaries and creates a bottleneck for innovation, impacting not just startups but also established players. Think of a seasoned project manager, frustrated as his team struggles to meet deadlines, not due to lack of ideas, but lack of skilled hands.
And then there’s the ever-present shadow of Ethical Concerns. As AI systems become more powerful, questions around bias, privacy, and transparency are becoming deafening. We’ve seen algorithms that perpetuate discriminatory lending practices or facial recognition software that misidentifies people of color. This isn’t just a technological challenge; it’s a moral one that demands careful consideration. A CEO, nervously watching the news cycle, now knows that a bad ethical choice is not just a bad look; it’s a recipe for disaster.
Actionable Insights: Mapping Your Course
So, what can strategists do? Firstly, embrace the democratization trend. Invest in platforms that empower a wider talent pool, not just highly specialized experts. Think training programs, citizen science initiatives, and collaborative workspaces. Secondly, lean into the open-source ecosystem. Encourage your team to contribute, build on shared resources, and cultivate a culture of knowledge sharing.
To mitigate the talent crunch, consider strategic partnerships with universities and invest in continuous learning programs for existing employees. Build a compelling employee value proposition that highlights your company’s commitment to ethical data practices, creating an environment where top talent wants to be. Finally, be proactive with ethical considerations. Implement rigorous testing protocols, seek diverse perspectives, and prioritize transparency in your AI systems.
The Data Science landscape is a thrilling, complex place – full of both magnificent opportunities and treacherous pitfalls. By understanding these underlying forces, by embracing the positive and diligently addressing the adverse, companies can not only survive, but thrive, in this data-driven age. This isn’t just a market; it’s a future we’re shaping together, one line of code, one insight, at a time.
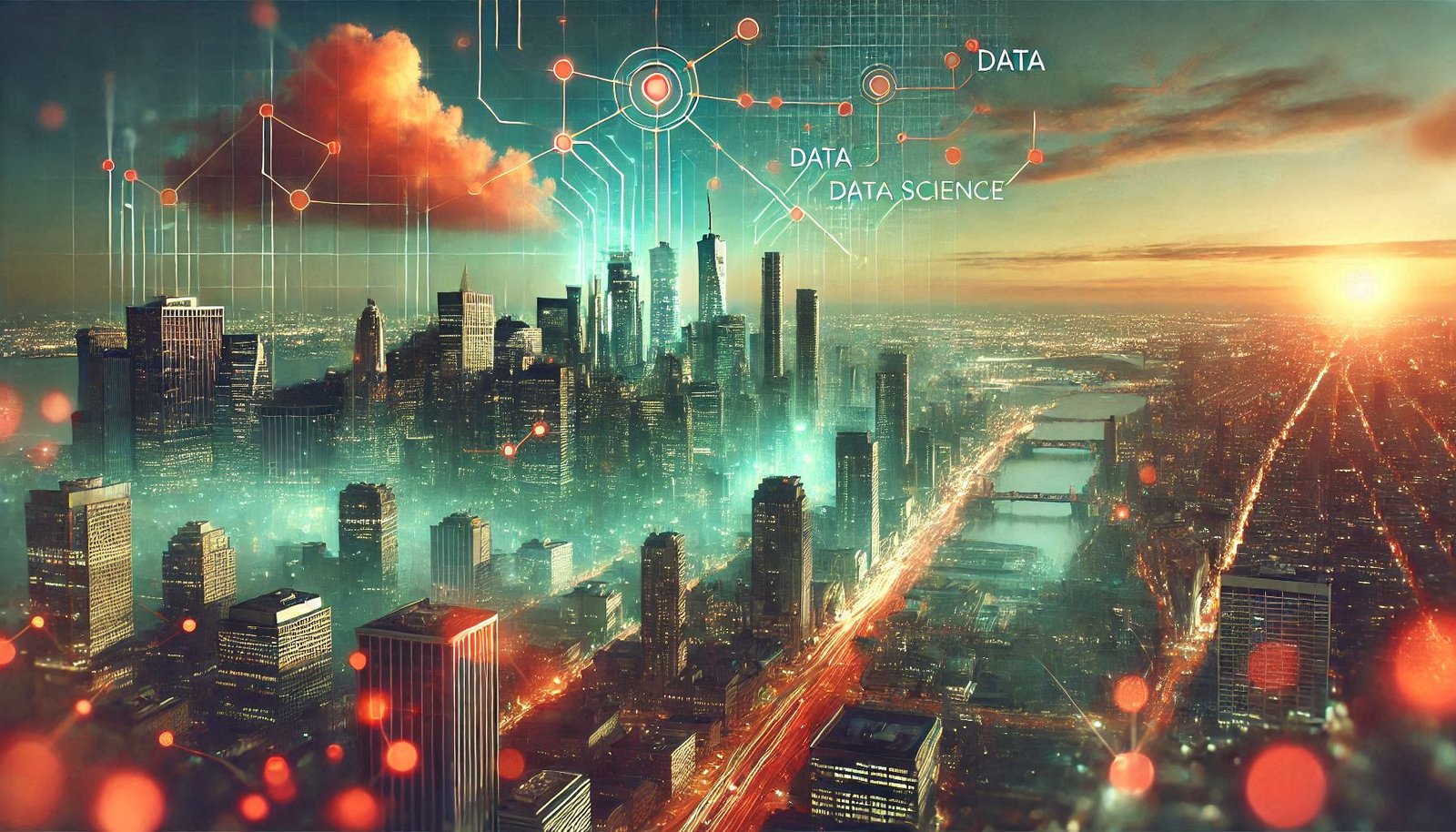
Healthcare: Imagine a bustling hospital, its fluorescent lights humming above. Dr. Ramirez stares at a complex patient file; a young woman with a rare genetic disorder. It’s like navigating a labyrinth with only a flickering candle. But here’s where data science steps in. A predictive model, trained on thousands of similar cases, illuminates the path. Suddenly, the labyrinth becomes less daunting. It highlights the most effective treatment options, reducing the guesswork and saving precious time. The model doesn’t replace the doctor’s expertise, but it augments it, guiding them towards quicker, more accurate diagnoses and personalized treatment plans.
Technology: Picture a slick online retailer, overflowing with a kaleidoscope of products. The website is a constant hum of activity – clicks, scrolls, and abandoned carts. But nestled in this digital chaos is a data science engine, working tirelessly. It’s like a seasoned detective, analyzing every user interaction to reveal hidden patterns. It can predict the products a customer is most likely to buy and instantly tailor the website to their unique tastes. Recommendations pop up like gentle whispers, “You might also like this,” or “This one’s trending now,” leading to increased sales and a personalized customer experience.
Automotive: Step into a futuristic automotive factory, where robotic arms dance with precision. Data streams flow like rivers, carrying insights on production line efficiency. A data science model, like a keen observer, detects minute anomalies – a slight dip in paint application, a subtle vibration in an engine assembly. It sends out warnings, like urgent telegrams, to the manufacturing team. These tiny tweaks, flagged by the model, add up to massive improvements in efficiency, reducing defects, minimizing waste, and ensuring each vehicle rolls off the line at peak performance. It’s not just about fixing problems; it’s about achieving perfection, one data point at a time.
Manufacturing: In a sprawling, smoky steel mill, the roar of machinery echoes through the halls. A seasoned foreman, hands calloused from years of work, watches as molten metal is poured into molds. Data science works like a silent, unseen partner here. Predictive maintenance algorithms constantly monitor the health of machines, listening for the first signs of trouble. It’s like having a team of tireless sentinels on duty all the time. When a critical part is close to failure, it alerts the foreman, allowing for proactive repairs before expensive breakdowns. The clanging of hammers and the hiss of steam are now accompanied by a quiet hum of efficiency, optimizing operations to new heights.
Okay, here are some key strategies employed by companies in the Data Science space since 2023, structured in a narrative way, aimed at Data Science professionals and IT leaders:
The Beginning: Laying the Foundation
The first move we saw was a significant push towards democratization of AI tools, often through low-code/no-code platforms. Think of a company like DataRobot, pivoting hard, focusing on platforms that allow even business analysts, not just dedicated data scientists, to build and deploy models. This lowered the barrier to entry, allowing for wider adoption across departments and faster experimentation cycles. This shift was organic, born out of the need to scale data science efforts across larger teams, making it less of a niche activity.
The Middle: Expansion and Consolidation
Then came the acquisition spree. Inorganic growth became a common tactic. For instance, a company like Databricks, known for its lakehouse architecture, started acquiring smaller players focused on specific AI capabilities like ML model monitoring, or specialized data labeling. These acquisitions weren’t just about adding headcount; they were strategically aimed at completing the puzzle, creating more comprehensive suites of data tools under one umbrella. One of the leaders at Databricks was heard saying, “It’s about a complete data journey now, not just point solutions.” This speaks volumes about the consolidation we’ve witnessed.
The End: Focus and Specialization
The final strategy, somewhat a reaction to the consolidation, was a sharper focus on niche solutions. Some companies, instead of trying to be everything to everyone, specialized in specific verticals. For example, smaller startups emerged offering very tailored AI solutions for healthcare diagnosis or supply chain optimization, where they became experts in unique datasets and modeling requirements. This organic growth saw smaller, more nimble players out maneuvering larger players in specific areas, proving that ‘focus’ is not to be forgotten amid a market looking for comprehensive solutions. The shift saw these companies winning deals over larger competitors for their superior tailored knowledge.
Here’s a draft of the Outlook & Summary section:
Outlook & Summary: Navigating the Data-Driven Horizon
If the last decade was about the explosive growth of data, the next five to ten years will be about its masterful orchestration. Imagine, not just rows and columns of information, but a symphony of insights conducted by the virtuoso of data science. We stand at the precipice of a truly transformative era where IT, no longer just the backbone, becomes the very beating heart of innovation, powered by intelligent algorithms and predictive models. This isn’t a gentle ripple, but a tidal wave. Picture bespoke systems adapting in real-time, anticipating user needs before they’re even articulated. The silent revolution we’ve explored throughout this article isn’t just about faster processing; it’s about crafting experiences that feel intuitive, almost magical.
The key takeaway isn’t simply that data science is important; it’s that it’s the force reshaping the very fabric of IT. It’s the sculptor molding raw information into actionable strategy, the alchemist transmuting data into competitive advantage. This means that IT professionals aren’t just managing servers; they’re becoming architects of the future, and business leaders are evolving into visionaries guided by data-driven clarity. We’re leaving behind a world where technology simply supports, and entering a realm where it propels, anticipates, and even dreams. The old rules are being rewritten, and the ink is still wet.
So, as you reflect on the quiet revolution unfolding, are you ready to embrace data science not just as a tool, but as the very lens through which you envision the future of your IT and indeed, your business?